Integrating AI to support manufacturing operators, not to replace them.
Integrating AI solutions in processes is getting more and more popular among manufacturers. According to a recent report by Capgemini (1), more than half of the European manufacturers (51%) are implementing AI solutions, with Japan (30%) and the US (28%) following in second and third.
COVID-19 has also accelerated AI adoption in the industry, as we have seen in many other sectors (you can find our analysis on COVID-19’s impact on the Public Sector here). In the manufacturing sector case, this is also evident in the Google Trends graph below, which shows a spike in searches for “AI in manufacturing” at the start of the pandemic. Google Trends shows a largely unfiltered sample of actual, anonymous search requests made to Google.
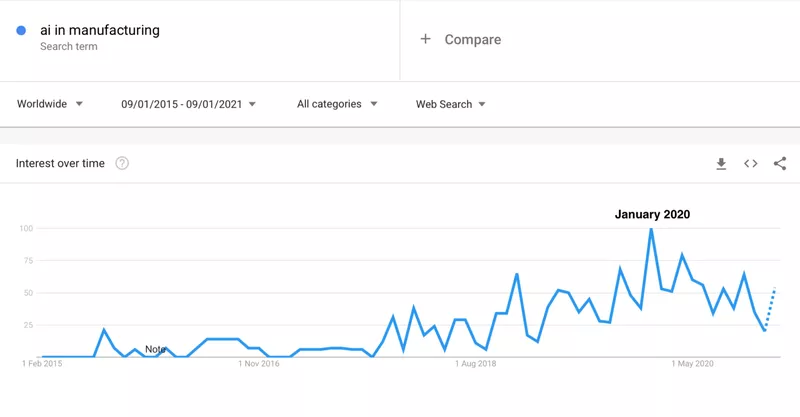
Source: Google Trends
While AI is now a differentiator for manufacturing, it is predicted to be standard operations soon. In fact, AI in manufacturing is predicted to grow by more than 50% annually through 2027. This is also due to many manufacturers with fewer than 50 employees jumping in (2).
So, how do you make sure you stay ahead of the curve to grow fast and boot profits? This article will cover how manufacturing companies can get started and scale with AI, providing examples from our experience and the broader landscape. We will also make a case for AI that supports manufacturing operators, not replace them.
What does integrating AI in manufacturing look like?
When it comes to integrating AI into manufacturing processes, there are three choices:
You outsource it. You work with expert partners that help you accelerate adoption by bringing in deep expertise and experience. This is often the most cost-efficient option in the short term.
You do it yourself. You internalize the project and build up a capability that will be useful for future projects. You also have greater control over your setup.
You collaborate with trusted partners. Partners will advise you and give you a fresh outsider perspective on your problems and leverage their expertise across clients and industry. Your data science team is involved all along.
To read more about getting off to a good start with AI, read our blog “Five keys to set your AI project up for success”.
Show me an example of a successful AI project in manufacturing
At Superlinear, we work with our clients in a close partnership. We believe in the combination of three elements for a successful implementation: business, people and AI.
Business: you need a specific and measurable problem with a clear ROI
People: delivering technology that can truly assist people, not replace them
AI: automating intelligence to achieve the highest impact
Let’s look at how this approach worked for our client Atlas Copco.
Atlas Copco is a global industrial company and world leader in air compressors manufacturing. Production planning on the assembly lines is crucial for the company’s activities, allowing the transmission of accurate and reliable information to employees and clients.
They approached Superlinear with the goal of optimizing the production planning of Atlas Copco’s Portable Air manufacturing sites, where complex products are assembled to fit its customers’ requirements. The project aimed to make production planning faster, more efficient and accurate while reducing human time spent and improving the number of orders delivered on time.
A clear return on investment
The project had a clear ROI: Superlinear’s AI-powered optimizer helps save the human planner 91.6% more time, improves the number of orders delivered on time by 9.0%, and reduces the total number of days orders are late versus the first promised delivery date by 17.2%. Our AI solution is also built with 100% open-source software.
In addition, our technology makes life easier for Atlas Copco’s employees by allowing both Production Planners and Sales Engineers to generate accurate and optimal production plannings quickly.
By bringing together business, people, and AI, our collaboration with Atlas Copco is a fruitful one. Our solution is currently implemented at two Atlas Copco production sites in Belgium and one in the USA.
Learn more about our project with Atlas Copco.
Integrating AI to support manufacturing operators, not to replace them
According to McKinsey (3), manufacturing companies have not kept up with the latest advances in analytics and in decision-support solutions that apply AI compared to other sectors.
This is because manufacturing operators still rely on their experience, intuition, and judgment. McKinsey brings the example of how today’s downsized teams of control-room operators are expected to monitor a multitude of signals on numerous screens manually and adjust settings as needed. At the same time, they must troubleshoot and run tests and trials, to name just a few of the tasks that strain the limits of their human capacity. As a result, many operators take shortcuts and prioritize urgent activities that don’t necessarily add value.
By introducing AI in manufacturing processes, companies can support their employees by automating and speeding up complex tasks, allowing them to focus on more valuable activities.
In the following section, we will look at four use cases from the industry where AI can support operators' daily activities in a manufacturing environment.
Four popular AI use cases for manufacturing
1. Predictive maintenance
According to Capgemini (1), 29% of AI implementations in manufacturing are for maintaining machinery and production assets. Capgemini's research team found that predicting when machines/equipment are likely to fail and recommending optimal times to conduct maintenance (condition-based maintenance) is the most popular use case of AI in manufacturing today.
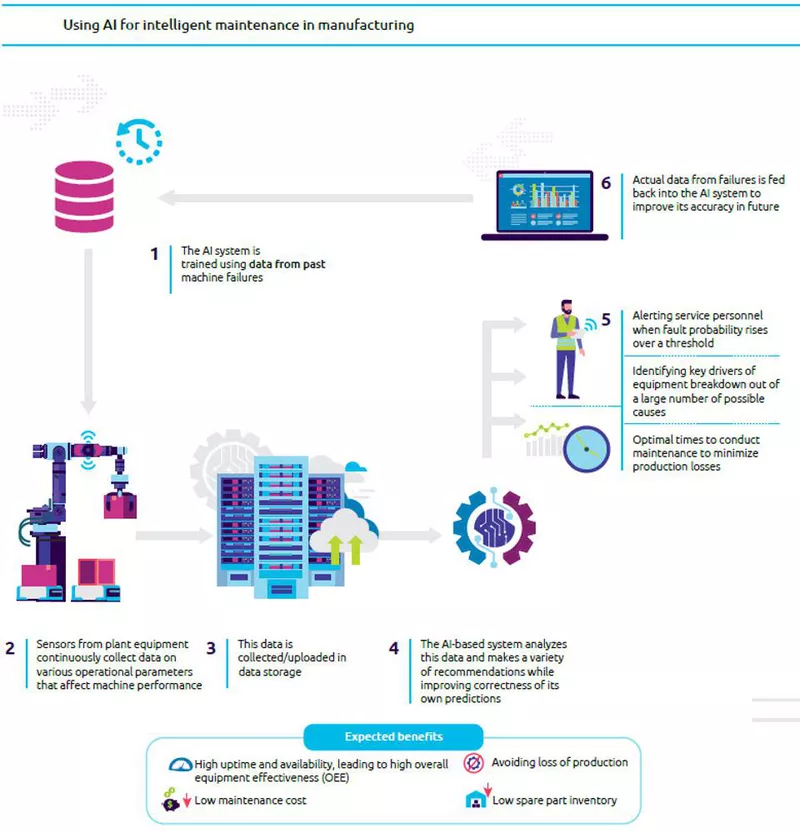
Source: Capgemini Report
2. Find early indicators of potential quality issues
AI can alert companies to problems in the production line that can result in quality issues. These faults could be major or subtle, but they all influence the overall level of production and could be eliminated in the early stages. AI can help operators assure quality on the assembly line.
For example, operators can detect the quality of materials or products using computer vision, which can then trigger an anomaly detection model to recognize when something is not normal. At Superlinear, we have applied computer vision in a similar way to detect bacteria in petri dishes for GSK. Read more in our case study.
3. Generative Design
AI can help organizations design products. A designer or an engineer inputs design goals into generative design algorithms. These algorithms then explore solutions and generate design alternatives (4). With machine learning, designers and engineers can test each iteration and improve upon it.
4. Knowledge management for service engineers
AI can help service engineers diagnose issues faster, identify the optimal solution, and avoid time and resources wasted due to machine downtime. Through a knowledge management solution, the engineers can look up the information they need in historical machine logs or technical documentation, so that the issues can be resolved faster.
Conclusion
To stay ahead of the curve, manufacturers should integrate AI in their processes to support their employees. As we have seen, the combination of business, people, and AI results in successful AI projects which deliver impact for years to come.
We also covered a few use cases to get started with AI, but of course, there are many more. Need help discovering what use cases could be most impactful for your manufacturing business? You can book a call with our Account Executive Tomas Vanhaeren to learn more.
Sources