The race to unmask the celebrities on VTM’s ‘The Masked Singer’ is in full swing, and joining the human sleuths this season is ONTMASKATRON, an AI-powered detective developed by Superlinear in partnership with Lieven Scheire. After making its debut last year, the AI is back and better than ever, operating in its enhanced form as ONTMASKATRON 2.0. How many singers has the AI correctly identified so far, and who are its current top suspects for the remaining masked singers?
AI joins the hunt
The concept of using AI to participate in the challenging guessing game of ‘The Masked Singer’ might have seemed far-fetched not too long ago, but it became a reality last year when Superlinear collaborated with Lieven Scheire to bring ONTMASKATRON to life. As Lieven Scheire explained, “Artificial intelligence excels at recognizing patterns. If every person has a distinct pattern in their singing and speaking voice, AI should be able to pick up on that.”
Superlinear’s team took on the challenge, creating ONTMASKATRON to compare the masked singers’ performances to potential suspects. However, the process was far from straightforward.
The AI must be able to handle a handful of challenges:
Making comparisons between singing and speaking voices
Navigating both Dutch, French, and English languages
Making sure data of all suspects are available to base its guesses. If no viewer suspects the right candidate, chances are there is no audio available for ONTMASKATRON to compare to.
It also lacks additional knowledge about the contestants’ backgrounds, the tips provided during the show, and whether or not someone is a professional singer, adding an extra element of intrigue.
ONTMASKATRON 2.0: What’s new?
Since last year, AI technology has advanced, and ONTMASKATRON has undergone significant updates and improvements.
As Superlinear’s expert Robbe explains: “With ONTMASKATRON 2.0, we’ve enhanced its pattern recognition and learning capabilities. It now processes data more efficiently, making it better at narrowing down suspects, even with complex inputs like duo acts.”
But how does this new version of ONTMASKATRON work, and what’s behind its advanced capabilities?
Behind the Magic?
At its core, ONTMASKATRON relies on Speaker Identification technology. This season, the AI is powered by ReDimNet, a state-of-the-art neural network introduced in 2024. Yet, the process isn’t as simple as plugging in the model and getting answers. Each step requires meticulous attention to detail to make sure the AI identifies the right celebrity.
Step 1: Gathering Clean Data
Gathering high-quality data is a common challenge in many real-life and business problems, and this case is no different.
On one hand, we need to source voice clips of each suspect from platforms like YouTube. These clips need to be as “clean” as possible—free from background noise, music, or interruptions.
On the other hand, we must enhance the singers' performances so that our model can process them accurately. This involves using a Music Source Separation Model (such as Demucs from META) to isolate the vocal track from the original music. After that, we manually refine the audio by removing sections where the singer is straining their voice, where jury members are speaking, or where backup vocals are present. Leaving only the clear, natural-sounding voice of the singer.
Step 2: Overcoming Model Biases
Even with the enhanced data, a significant challenge remains: the Speaker Identification model embeddings are biased. When attempting to match masked singing performances to potential suspects, the model often leans toward suspects who match the language of the performance or whose reference voice clips include singing. For example, if the performance is in English, the AI might favor English-speaking suspects, even when that isn’t necessarily correct. To address this, we apply Singular Value Decomposition (SVD) to break down and analyze the audio, isolating and removing dominant characteristics like language or singing style. This ensures that the AI focuses on vocal traits intrinsic to the speaker rather than external factors.
Step 3: Late Interaction Scoring
After refining the embeddings, ONTMASKATRON ranks each suspect for each masked singer. For this, we draw inspiration from the rapidly advancing field of generative AI. Instead of using the standard cosine similarity between the masked singers' and suspects' embeddings (known as ‘no-interaction’), we apply the concept of ‘late interaction’, a technique popularized by ColBERT for efficient and accurate retrieval in Retrieval-Augmented Generation applications. This allows us to more effectively match masked singers with suspects.
Unlike traditional embedding-based scoring, where entire audio fragments are compared, ONTMASKATRON breaks each audio clip into smaller snippets, comparing these directly during scoring. The full audio clips never generate their own embeddings. Instead, for each snippet of the masked singer's performance, we identify the snippet in the suspect's audio that has the strongest interaction with it. We then sum these interaction scores to produce a final ranking.
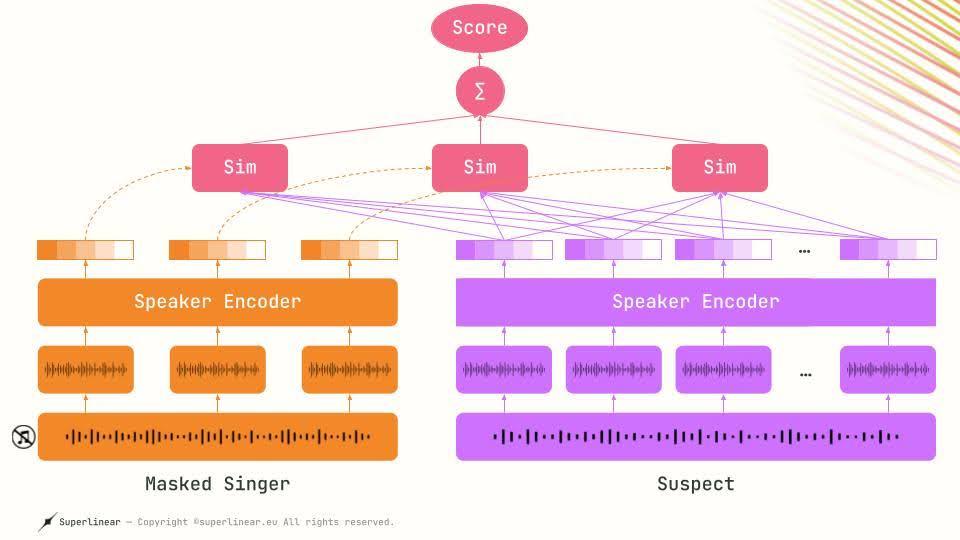
Duo complications
ONTMASKATRON faces additional challenges this year with duo performances. To address this, the AI now analyzes the voices of celebrity pairs as a whole, rather than individually. For example, instead of pairing Jani Kazaltzis with Dominique Van Malder, it correctly matches Jani Kazaltzis with Otto-Jan Ham, and Joris Hessels with Dominique Van Malder.
Results so far
Out of the contestants unmasked so far, ONTMASKATRON has correctly identified four out of five—a promising track record, especially given the complexities of analyzing performances with limited data. With every new episode, ONTMASKATRON’s accuracy improves as it gathers more data and refines its predictions.
What’s next?
As the show progresses, ONTMASKATRON 2.0 will continue to evolve and fine-tune its predictions. However, to keep the suspense alive, the AI’s conclusions for the remaining masked singers are being kept under wraps. But stay tuned—ONTMASKATRON is sure to surprise us with its next reveal.
Follow ONTMASKATRON’s journey and updates on X.com (formerly Twitter), where we share our AI’s latest insights and predictions.
Spoiler alert: Want to know who our suspects are? Make sure to check out this article.