We have been helping a number of corporate companies to kickstart their Data Science and Machine Learning activities and team. These are our lessons learnt.
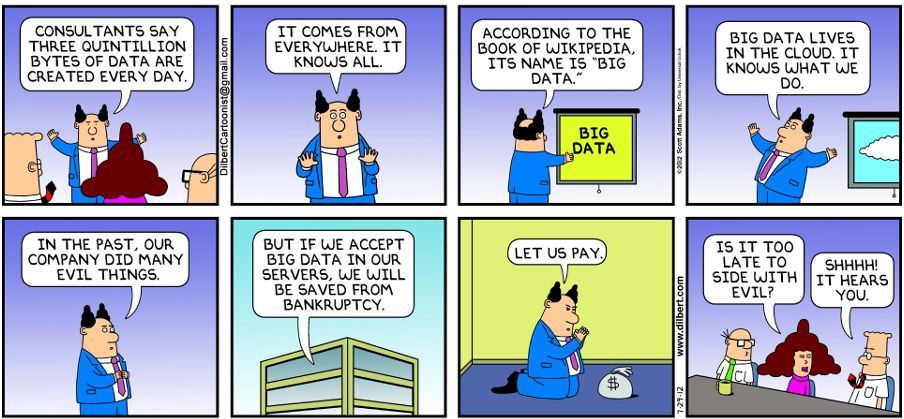
Our customers asked us to help them with identifying the right technologies, setting up initial Proof-of-Concepts, assembling a team and designing their overall data strategy and architecture.
The initial requirements are usually similar:
a roadmap of projects,
a Data Science platform,
a Data Science team, and
an initial set of Proof-of-Concepts.
However, we’ve observed (completely) different strategies to get Data Science embedded in their organisation.
It ranges from total centralization and isolation from their business to absolute decentralization and diffusion with business.
This is what we’ve learnt:
1. Neither full centralization, or decentralization work:
A centralised approach works to achieve results quickly, but we noticed that business engagement could have been better.
A decentralised strategy by first talking to the different stakeholders and business lines to get them on board as drivers. In the long run this creates support, but it takes time and can create redundancy.
We think you need a combination of both. Centralization for executing the projects and sharing Data Science knowledge and decentralization for a short connection towards business.
It is essential that business understands what the Data Science team is doing and vice versa. For example by embedding data scientists directly into product teams while still requiring them to report to a common director of data science.
2. Put your cleaned data on the Data Science platform use case by use case:
The danger of migrating all your data to a new platform before having clear use cases is that your budget drains out before you’ve created any added value.
To ensure business and management buy-in and avoid burning money on the wrong things, you need to migrate one use case at a time.
3. Before scaling your Data Science team, have a validated roadmap, management buy-in and an initial Proof-of-Concept or prototype.
It’s tempting to start hiring and scaling your Data Science team, but as long as there are no real use cases they will lack focus and direction.
Making sure Data Science is supported throughout the organization is your first goal. For this you need experts that can translate your business into Data Science or Machine Learning solutions.
4. Get your data stakeholders involved and give them dedicated time: owners, suppliers and users.
Working on Data Science projects requires input from a lot of people. If they’re not properly involved there’s a risk that they become bottlenecks. This creates overhead and delays.
Owners: they own the data source, but are not necessarily the end users. They can provide you an understanding of the data and the data model.
Suppliers: the people who are literally going to supply the data. It’s key that they have dedicated time to supply the data. They are often over demanded and this is just another request on their pile.
Users: the ones that are going to use the output. Whatever you build, it’s your end user that is going to tell you what.
5. Start from the data you have today for an initial Proof-of-Concept or prototype.
In every Data Science project data will be the hardest resource to make available. It will be locked away in some legacy systems, managed by overly questioned people.
6. Identify a roadmap with a series of workshops with business, having one specific use case as input.
Starting with a initial small use case as input makes that they can relate, understand the value and translate it to their situation.
We’ve seen that a basic understanding of the potential of Data Science is essential to have efficient workshops. Explaining Data Science with use cases that are hard to relate to, makes it more difficult.
7. Score on value vs. feasibility (both technically, data availability and politically).
The number of interesting Data Science projects is endless, but there’s an important trade-off between the feasibility and value.
When starting your Data Science activities it’s good to have a focus and some quick wins to convince everyone of the value. This requires to focus on the low hanging fruits.
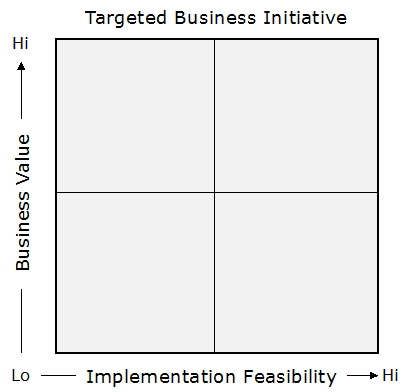
8. Start with a prototype.
Before fully launching anything, start with a prototype that has the least amount of features to deliver the basic value proposition. This enables you to gather end user feedback and creates value from the start. Then continue to iterate and improve, both technically and qua features.
We’ve a number of companies launching a complex and feature-rich product, but lacking any end user feedback. Eventually they had to strip down to the basic value proposition and start from there.
Keep in mind, your solution will never be finished. The data, technology and business requirements will keep evolving.
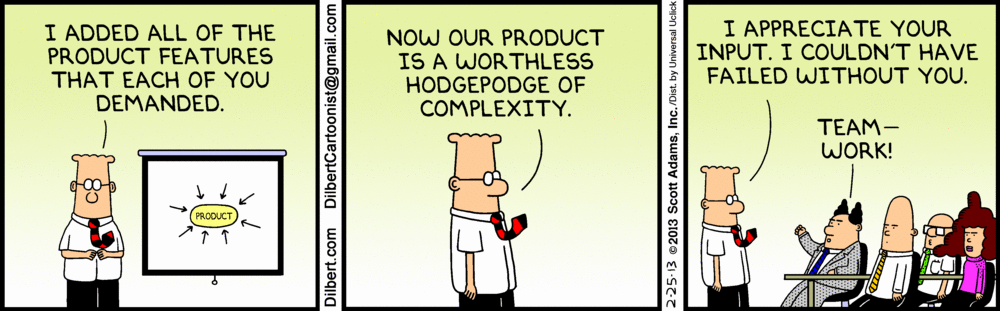
Conclusion
Work use case per use case with the bigger picture in mind, involving business and data stakeholders from the start.