The printing industry is undergoing rapid changes: printing companies need to continue to innovate to reduce costs, decrease production time and waste, and stay ahead of new trends. Especially increasingly shorter lead times and reduced order quantities disrupt production.
Like most industries today, printing businesses also have to deal with a heavily disrupted supply chain, impacting delivery times and the war for talent, pushing them towards approaching new solutions that can offer higher flexibility and agility.
In this article, you’ll explore why Scheduling in the Printing Industry is complex and how CERM, with the help of AI specialist Superlinear, addressed these challenges.
How print companies deal with modern scheduling complexities today
Printing companies typically allocate one or more staff member(s) to schedule production jobs. Jobs consist of multiple steps, each of which needs to be assigned to a machine (e.g. a press, cut, rewind etc.) and scheduled at a certain point in time, respecting various constraints and sequenced in such a way that products are ready for on-time shipping.
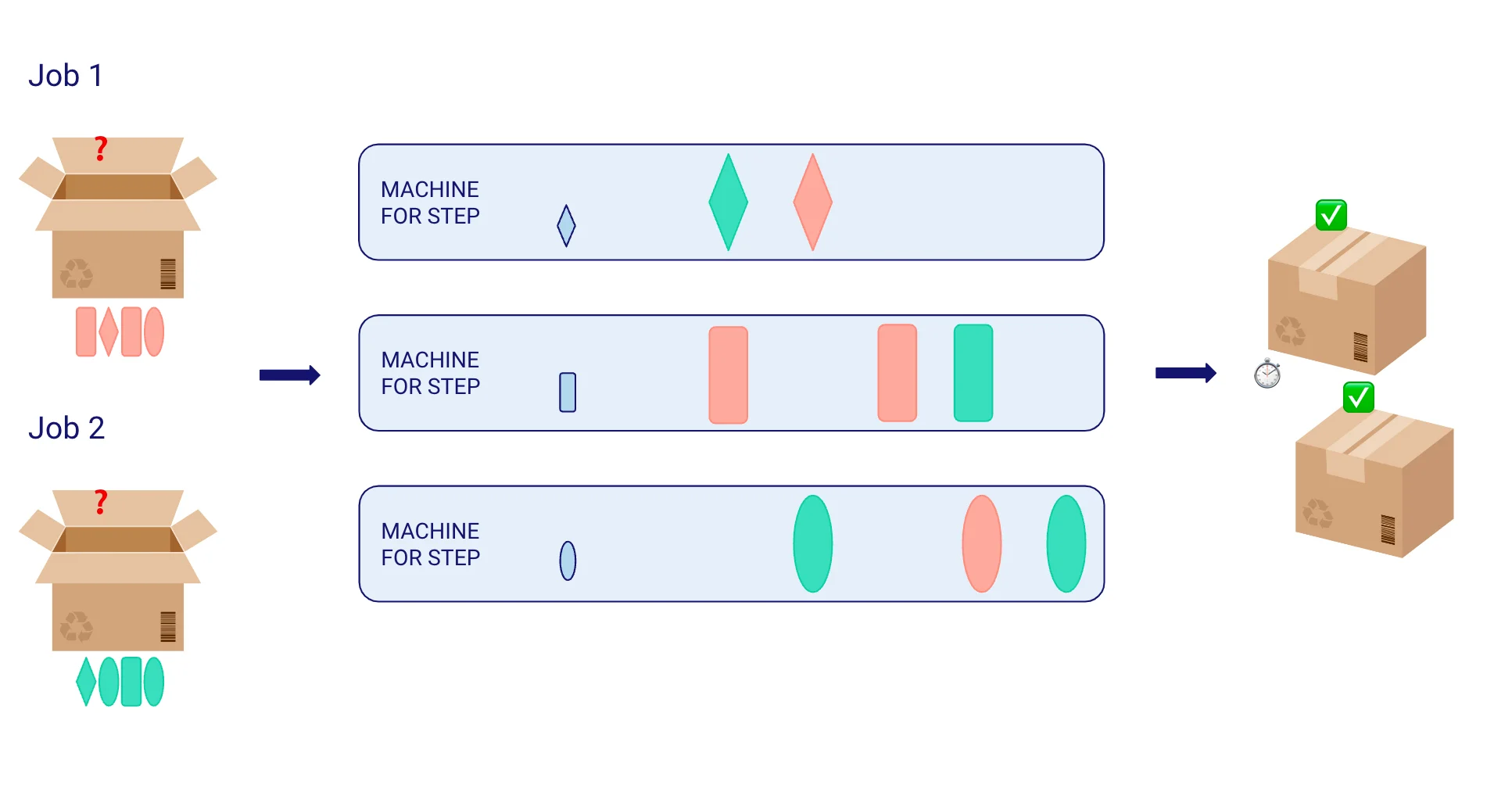
Scheduling production jobs is a time-consuming task and challenging to automate fully. CERM, a software development company providing solutions for labels and packaging printing companies, decided to take on this challenge with an innovative mindset.
CERM offers a complete end-to-end software solution covering the entire value chain of their clients, from prospecting customers, estimating prices, and product management up to creating the correct sales order and managing the production:

To tackle the complexity of modern scheduling, CERM contacted Superlinear, a Belgian Artificial Intelligence agency, to help develop new functionality for their clients' production scheduling module.
Superlinear, in collaboration with prof. dr. Seppe vanden Broucke (UGent), worked together with CERM to develop a bespoke cloud Artificial Intelligence (AI) engine which could be offered to all CERM clients, both improving their production schedule and reducing the manual (human) efforts to schedule.
What makes scheduling for the print industry planning so difficult?
In the field of operations research, the problem of optimally scheduling a bunch of tasks over devices is known as ‘Job Shop Scheduling’. It is a classic, well-studied problem with many techniques to solve it. However, the issues in optimizing print production schedules reach beyond the well-defined Job Shop Scheduling problem and make the task particularly challenging.
1. A multi-objective problem
When creating a production schedule, printing companies need to balance different tradeoffs. Is it preferred to have one order delivered ten days late, or would two orders each five days late be better? Perhaps it depends on the customer… If my scheduling operator has fixed a job on a machine at a given time, how many minutes/hours can this still be ‘moved’ to create a more optimal schedule? They would perhaps not mind delaying the job for half an hour to save 15 minutes of setup time…
Saving change-over time between jobs is crucial to reduce the total production time. But this time largely depends on the setup of the preceding job on the same machine. On a printing press, having two consecutive jobs with the same paper type and size will reduce the setup time of the second job (as the paper can remain in the press). Human scheduling will typically focus on improving setup efficiency as much as possible by sequencing jobs based on their technical characteristics (the same paper, die, inline-finishing, …). So the problem is a multi-objective one.
"Since automatic scheduling is new to all our customers, we wanted to offer them as much flexibility as possible to experiment with these tradeoffs"
Geert Van Damme, Managing Director of CERM
2. Many interacting constraints
A schedule is only helpful if it is valid. For example, executing two or more jobs on the same machine simultaneously is not feasible in print production. Still, some manually created schedules overlap by mistake due to the number of steps to be planned and the constant flow of new orders coming in. This is a clear sign of information overload for the human scheduler.
But apart from overlaps, many other constraints need to be respected:
You cannot execute all steps on all devices. Typically, you choose the cheapest production device before scheduling. But there might be alternatives available. This is the flexible version of the ‘Job Shop Problem’. But what is uncommon is the preference for the initial (cheapest) choice: you would like to produce a 4-color job on a 4-color press, but the five and the 8-color press could also be used.
The total production time is related to the sequence of jobs. Typical ‘Job Shop’ adaptations include setup time based on the machine. But in Print production, the setup time is also related to the preceding job on the machine because some settings might be identical. So changing the sequence of jobs changes the total production time, uncommon to ‘Job Shop Scheduling’.
The work schedule of machines may vary daily, and jobs will sometimes be stopped and continued some hours later (e.g. the next day). Print production's ‘preemptive’ character is more complex to solve than a straightforward 24/7 non-stop production.
Printing is only possible when resources are available. Paper inventory requirements define the earliest possible start of jobs and the maximum quantity that can be produced. At the same time, some of the resources, like die-cut tools, might become available again after production.
Roll-to-roll production with multiple jobs printed on the same roll will need consecutive steps to produce these jobs in the reverse sequence. Winding and unwinding reverse the sequence as long as jobs are not separated.
So technically, this particular version of the Job Shop Scheduling problem is multiple-objective, weakly flexible and weakly ordered with a sequence-dependent setup, pre-emptive tasks, capacity and inventory.
3. Working fast on a large scale
As an additional challenge, many clients of CERM are large printing companies, with hundreds of jobs containing multiple steps each over dozens of devices to be scheduled. This requires a robust and powerful computing infrastructure and well-optimized code.
On top of this, planners testing an AI-engine love to see a good first solution after only fifteen minutes of waiting, putting additional strain on the solver. This is not how the AI engine will be used in real life: 2 hours of computing will replace 6 hours of manual work. But instead of constantly changing the schedule, the operator will probably use 2 ‘optimizations’ per day and spend some more manual time to solve the emergencies.
Geert Van Damme adds :
"Printers will probably be happier if they can move away from constantly changing schedules. They will just need to learn to fix the next 4, 8, 12 hours and run optimization only a few times per day."
4. Explainability of optimized schedules
The final challenge is not technical but related to the acceptance of an automated approach. Planners can be very particular in their preferences or might look only at a local level, e.g. in case, every planner in a team is responsible for a set of devices. While testing the solution, they sometimes found the given planning “illogical” or “suboptimal”. Here, both Superlinear and CERM had to allocate sufficient time to provide explanations and fine-tune the objective tradeoffs of the solver.
One of the observations was that the objective of reducing setup time could, in some cases, come at a direct cost in terms of late orders. This was a surprising finding for the involved stakeholders and showed how AI techniques could sometimes lead to unexpected insights.
CERM’s response to the scheduling maze: a custom-built solution
Tools exist that try to offer ready-made solutions for Job Shop and related scheduling problems (factory floor production scheduling and school time-tabling are two common examples). However, these were not an option in this case for multiple reasons:
Most do not support the full range of constraints present here and hence would not be a perfect fit.
CERM would not be able to integrate such third-party tools into its software offering.
Some branche-specific software-tools implement “rule of thumb” scheduling approaches, designed for a standard set of production processes, not allowing the addition of a new production method or machine without programming.
More generally, one can also find open-source and commercial mathematical solvers for optimization problems. One drawback of these tools (apart from high licensing fees for commercial offerings) is that they require the user to express the problem in mathematical terms as a mixed integer programming (MIP) model. Such models can significantly increase development time and make it hard to implement changes or new requirements down the line.
To resolve these issues, Superlinear has developed an in-house optimization architecture which can run on top of these solver backends and allows to specify objectives and constraints in a manner which strikes a balance between flexibility and efficiency without being tied to one particular solver vendor.
However, in the case of CERM, we found that even the best available commercial MIP solvers could not deal with realistically sized problems CERM was facing. As such, Superlinear started to explore other approaches. Another category of available optimization tools tries to tackle highly complicated optimization problems by implementing so-called heuristic approaches (such as tabu search, genetic algorithms, etc.). These adapt more greedy techniques to search the solution space for a solution but typically come with tradeoffs regarding solution quality. Also, heuristic approaches usually have a hard time finding good solutions in case many hard (i.e. unbreakable) constraints are present, as is the case here.
Hence, Superlinear decided to take a different strategy and use a constraint, programming-based solver. This forms yet another category of optimization techniques and uses advanced search and AI techniques to find good solutions fast when confronted with a vast search space. In this case, Google’s open-source or-tools CP-SAT was found to excel in this task and was hence added as a backend “engine” to Superlinear optimization architecture. With careful tuning of the objectives and constraints and designing a multi-staged approach that solves the problem in multiple stages (first focusing on the primary goal, then gradually introducing secondary objectives), real-world planning could be tackled.
The solution was deployed as an API using Azure Batch to provide the backend compute infrastructure, guaranteeing scalability and ensuring we could allocate powerful instances to solve each incoming problem.
What’s next for scheduling at CERM?
CERM is currently testing the approach with different test customers, with initial results looking promising. Interestingly, the project also helped to reveal several data quality issues, which were subsequently resolved. In particular, customers are pleased that the time-consuming and exhaustive task of printing production planning can finally “be done by a computer.”
Scheduling operators will have to be convinced that the AI-optimized schedule will significantly help them focus better on the many day-to-day emergencies. So more test and simulation tools will be added in the coming months.
Geert Van Damme adds:
"Since operators need to give complete control to the optimization software, they need to gain trust by testing several days or weeks in a row".
But finally, this scheduling optimization tool will be improved, step by step, based on the experience of the CERM customers. More refined parameters will make it one of the most time-saving tools in the print shop, as well as for production and the human scheduler.